Pharma & Biotech
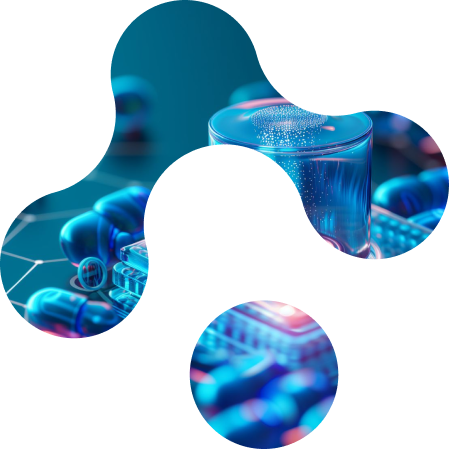
Harmonize
medical code lists across all phases
Build
semantic applications to automate data collection and reporting.
Unite
substances and trial data in a enterprise semantic layer to gain speed and insights
Our data-centric approach improves efficiency and eliminates silos in all phases of the R&D lifecycle.
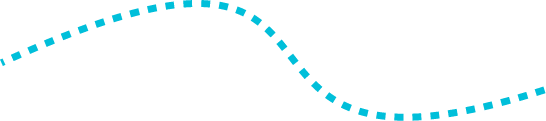
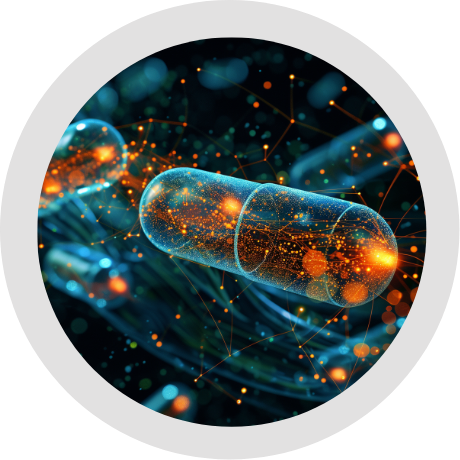
We help manage changing requirements due to evolving compliance rules and regulations, and new strategies.

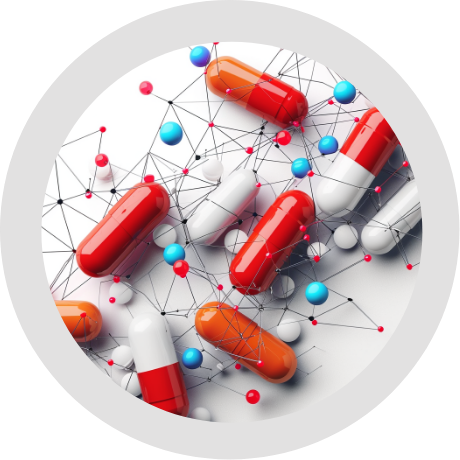
We can integrate data from third parties – including scientific journals, patent databases, CROs, CMOs, EMRs, regulators, or public Web content, as well as life sciences vocabularies such as SNOMED CT, ICD10, and MedDRA
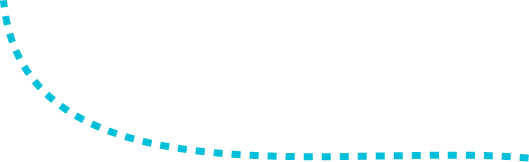
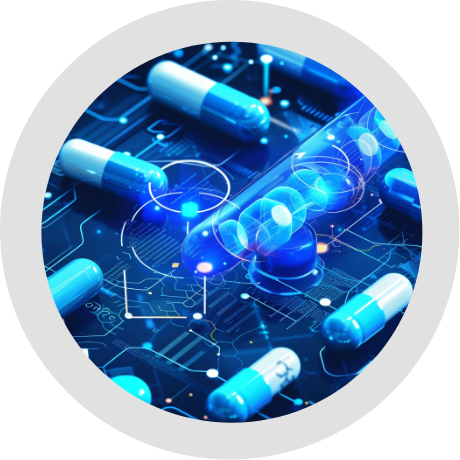
Harmonization
TopBraid EDG is the preeminent knowledge graph creation and curation tool. Knowledge graphs are flexible and powerful, and can be used to harmonize medical code lists such as MedRA and SNOWMED across all phases of the R&D lifecycle.Knowledge graphs are also inherently interoperable, and EDG supports many methods to maintain data quality, such as via federated governance. The resulting data products are highly reusable and can be leveraged to automate processes and reporting throughout the R&D lifecycle.
Build
Data products can be combined with other data assets and tools, to bring automation to important — and yet common — procedures in R&D, such as adverse event reporting. For example, a data product of harmonized internal code lists could be expanded on to update automatically when external code lists are updated.
Unite
By modeling the substances, processes, policies and data in clinical trials in a knowledge graph, you provide a holistic view of the entire R&D lifecycle and uncover insights from previous trials. These semantic layers can be built from the bottom up with an accumulation of semantic data products that work together, or from top-down initiatives such as a semantic data catalog or master data management initiatives.This enterprise semantic layer FAIRifies your data, making it easier to reuse and ultimately speeding product development. Enterprise semantic layer also improves the speed and effectiveness of AI initiatives, while also providing transparency and explainability into the results of the algorithms.
We can help make data more findable accessible interoperable reusable (FAIR)
The TopQuadrant
Difference
TopBraid EDG is the ultimate knowledge graph creation and curation tool, and was built to get the most out of the most expressive and limitless information architecture, RDF.
A fixation on time to value
20+ years of delivering business value through white glove partnerships and a platform built by pioneers in the semantic web, preloaded with accelerators, assistants, and over 300 ontologies to get you off the ground fast
Collaboration fit for the enterprise
Helping organizations define and find their critical data through centralized search, workflow versioning, and robust integrations for seamless insights across the enterprise.
Proving value every day
Solutions that are fully compliant with W3C standards to enable transparency and portability, assuring no vendor lock-in, allowing you to build safely and freely
Set the stage for innovation
Build the foundation for enterprise GenAI and LLMs, enabling secure, scalable data connections and empowering AI-driven insights and innovation.